Leveraging Self-Owned AI in Genetic Engineering
Learn how Genetic Engineering companies can leverage self-owned AI to enhance their operations and drive innovation.
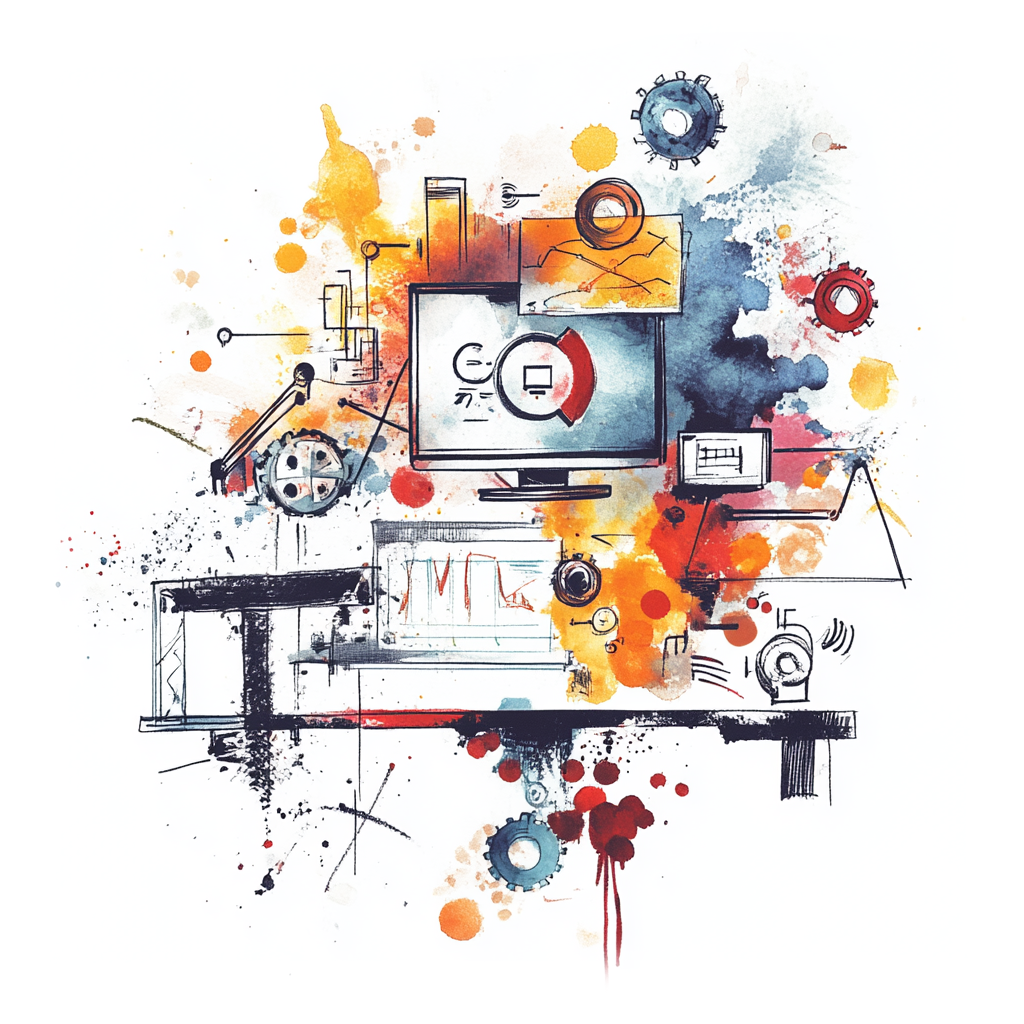
Unlocking Potential: How Upstream Operators in Genetic Engineering Can Utilize Their Own AI
In the evolving world of genetic engineering, upstream operators find themselves at the forefront of groundbreaking innovations. With a surge in data and scientific advancements, there’s a powerful ally waiting to be harnessed: your very own artificial intelligence (AI). Imagine having an AI that understands your specific needs, keeps pace with your research, and can help you make sense of the vast amounts of information at your fingertips. Let’s explore some of the remarkable ways that a self-hosted AI can transform the genetic engineering landscape.
Use Cases That Speak Volumes
1. Research Optimization
Picture this: you've got a team of researchers trying to identify the best gene-editing techniques for a new product. Your AI can analyze hundreds of articles, studies, and reports in mere seconds, showcasing trends and important findings. It can even suggest the most promising directions for further research. With AI by your side, you'll optimize research efforts and save countless hours of manual searching.
2. Data Management
In genetic engineering, data is your best friend—but it can also feel like a chaotic mess. Managing the huge volumes of data generated during experiments can overwhelm even the sharpest minds. A self-hosted AI can streamline this process, organizing and interpreting data sets, so you always know where your projects stand and what steps to take next.
3. Regulatory Compliance
Complying with regulations is critical in the industry. With local and international laws constantly changing, it’s hard to keep up. Imagine having an AI that keeps an eye on regulatory updates relevant to your work, ensuring that your projects remain compliant and minimizing any costly errors. Think of it as a compliance watchdog that effortlessly keeps you informed.
4. Predictive Analysis
What if you could anticipate project outcomes before investing time and resources? A personal AI can leverage historical data to build predictive models, allowing you to foresee success or potential hurdles in your experiments. This capability can make decision-making more informed, reducing risks, and accelerating innovation cycles.
The Benefits of Your Own AI
Investing in your own AI isn’t just about keeping up; it’s about gaining a competitive edge. Here are some compelling advantages that come with developing a self-hosted AI:
-
Customization: Unlike generic solutions, your AI can be tailored to your unique needs and research focus. It learns from your data and gets smarter as you work together.
-
Security: You control your data. Having your AI means sensitive information stays in-house, protecting your proprietary research and intellectual property from potential breaches.
-
Cost-Effectiveness: A self-hosted AI can save you time and money over the long haul. It can automate tasks that once required significant manpower, freeing your experts for higher-level initiatives.
-
Real-Time Insight: With your AI constantly processing new information, you gain access to real-time insights that can inform critical decisions without delays.
Steps to Get Started with Your Own AI
Now that you see the exciting possibilities ahead, let’s dive into how you can actually make this happen:
Step 1: Identify Your Needs
Begin by analyzing your workflows. Which processes could be streamlined? What type of data do you have, and how can it be optimized? Jot down specific goals you want your AI to achieve.
Step 2: Gather Your Data
Knowledge is power. Collect all relevant data that your AI will need. This includes experimental results, regulatory frameworks, research articles, and historical project data.
Step 3: Choose the Right Tools
Look into various open-source AI frameworks like TensorFlow or PyTorch that fit well within the genetic engineering field. The choice depends on the required level of complexity and your team's expertise.
Step 4: Team Up with Experts
If you don't have in-house AI capabilities, consider collaborating with data scientists or hiring AI developers. You’ll want a team that can help build and customize your AI system to your specific needs.
Step 5: Train Your AI
Your AI needs to learn from your data. Use the collected data to train your model, constantly refining it based on feedback and results. This is where the magic happens, as the AI starts to adapt to your preferences and operations.
Step 6: Implement and Iterate
Once your AI is up and running, it’s time to put it to work! Monitor its performance closely and make adjustments along the way. This is a continuous process of improvement, allowing you to get the most out of your investment.
With a self-hosted AI at your disposal, upstream operators in genetic engineering can take charge of their data, streamline processes, and unlock innovations that drive the industry forward. Embracing your own AI is not just a trend; it's a strategic move that could redefine your operations and elevate your research capabilities. Welcome to the future of genetic engineering!